IPG
An Integer Programming Game is a simultaneous game among \(n\) players solving a parameterized (mixed) Integer Program. In ZERO, we support IPGs where each player \(i\) solves the following problem:
Where \(\mathcal{I}\) is the set of indices of integer variables. In other words, the objective function takes a specific bi-linear form.
A quick example
Consider the following Integer Programming Game: The first player is the x player, whose decision variables are \(x\). The second player is the y player where its variables are \(y\).
Note
We slightly change the notation with respect to ZERO’s arguments for the exposition of this game. For instance, here for \(x\), we point to the first player’s variables instead of the parameters of a IP_Param
. The example below should clarify any doubt.
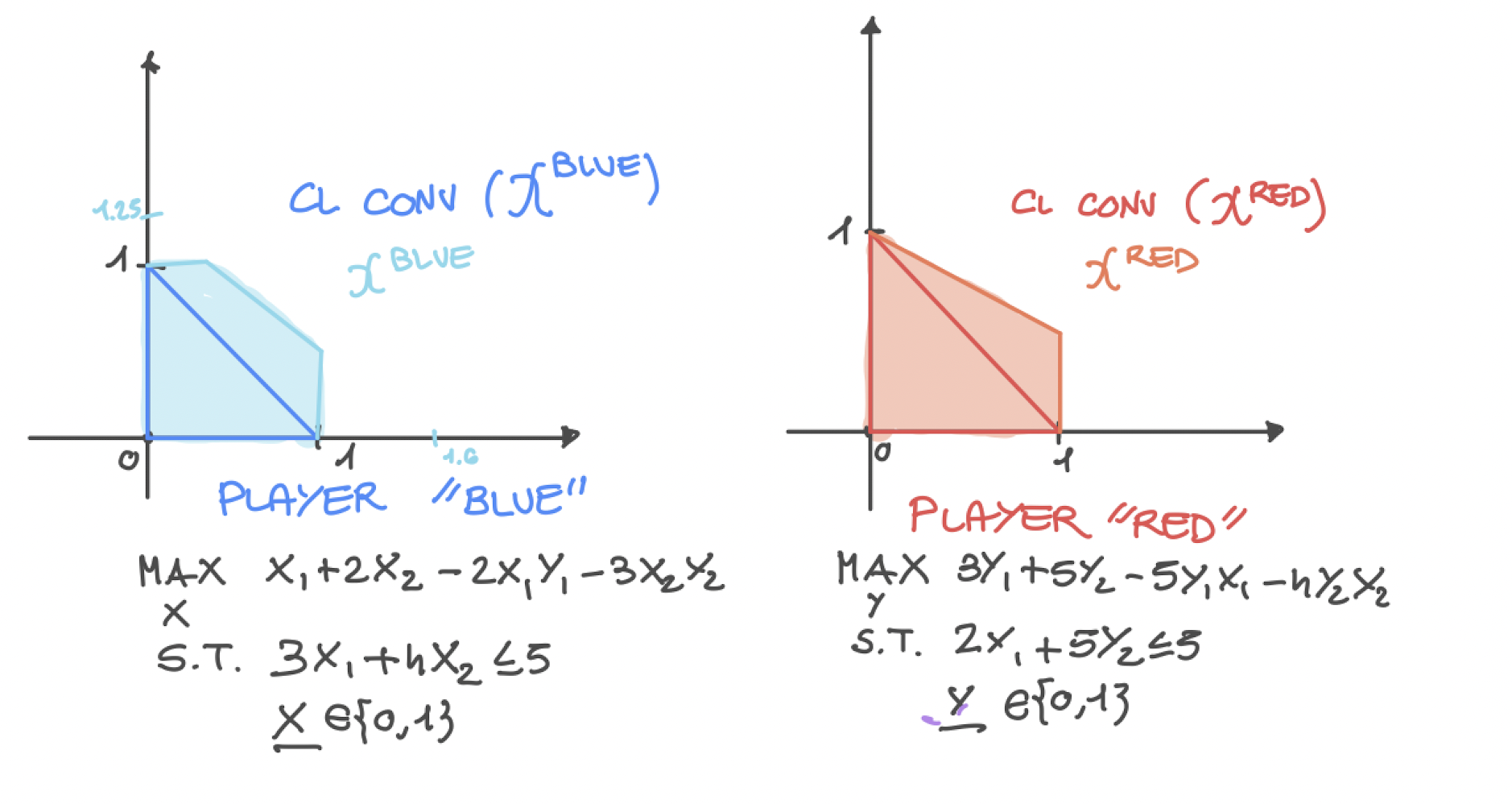
The x player’s optimization problem is as follows
While the y player’s optimization problem is:
The problem has two pure Nash equilibria \((x_1, x_2, y_1, y_2) = (0, 1, 1, 0)\), and \((x_1, x_2, y_1, y_2) = (1, 0, 0, 1)\), and a mixed Equilibrium \((x_1, x_2, y_1, y_2) = (2/9, 7/9, 2/5, 3/5)\).
Modeling and solving the problem
The first step in modeling this Integer Programming Game is to include zero.h and create a derived class of Game::IPG
. The minimal constructor for Game::IPG
involves passing a pointer to GRBEnv (Check Gurobi’s C++ reference manual
). The derived class should indeed instantiate the base class (Game::IPG) using such a constructor. The code below gives an example.
#include <zero.h>
int main(int argc, char **argv) {
GRBEnv GurobiEnv;
try {
Models::IPG::IPGInstance IPG_Instance; // The IPG Instance
int numItems = 2, numPlayers = 2;
arma::vec c(numItems), d(numItems * (numPlayers - 1)); // Profits c in the objective
arma::sp_mat C(numItems * (numPlayers - 1), numItems); // C terms in the objective
arma::sp_mat a(1, numItems); // LHS for Knapsack constraint
arma::vec b(1); // RHS for constraints
arma::vec IntegerIndexes(numItems); // The index of the integer variables
VariableBounds VarBounds = {{0, 1}, {0, 1}}; // Implicit bounds on variables
// Fill the values in the paramterized integer problem
for (unsigned int i = 0; i < numItems; ++i)
IntegerIndexes.at(i) = i;
C(0, 0) = 2; // C terms in the objective
C(1, 1) = 3;
a(0, 0) = 3; // Knapsack Constraints
a(0, 1) = 4;
b(0) = 5; // Knapsack Capacity
c(0) = -1; // The standard is minimization, hence minus
c(1) = -2;
d(0) = 0;
d(1) = 0;
// Create a parametrized Integer Program
MathOpt::IP_Param PlayerOne(C, a, b, c, d, IntegerIndexes, VarBounds, &GurobiEnv);
// Parametrized Integer Program for the second player.
C(0, 0) = 5;
C(1, 1) = 4;
a(0, 0) = 2;
a(0, 1) = 5;
c(0) = -3;
c(1) = -5;
MathOpt::IP_Param PlayerTwo(C, a, b, c, d, IntegerIndexes, VarBounds, &GurobiEnv);
// Add the players to the instance. We can also specify a file path to write the instance
IPG_Instance.addIPParam(PlayerOne, "A_Parametrized_KnapsackProblem1");
IPG_Instance.addIPParam(PlayerTwo, "A_Parametrized_KnapsackProblem2");
IPG_Instance.save("A_Knapsack_Game"); // Save the instance with the standardize format
Models::IPG::IPG KnapsackGame(&GurobiEnv, IPG_Instance); // Create a model from the instance
// A few optional settings
KnapsackGame.setNumThreads(4); // How many threads, if supported by the solver?
KnapsackGame.setTimeLimit(5); // Time limit in second
KnapsackGame.finalize(); // Lock the model
KnapsackGame.setDeviationTolerance(3e-4); // Numerical tolerance
// Run and get the results
// Cut and Play
KnapsackGame.setAlgorithm(Data::IPG::Algorithms::CutAndPlay);
KnapsackGame.setLCPAlgorithm(Data::LCP::Algorithms::MIP); // How do we solve the LCPs?
KnapsackGame.findNashEq();
std::cout << "The Cut-and-Play solution" << std::endl;
KnapsackGame.getX().at(0).print("Player 1:"); // Print the solution
KnapsackGame.getX().at(1).print("\n Player 2:");
// Zero Regrets
KnapsackGame.setAlgorithm(Data::IPG::Algorithms::ZERORegrets);
KnapsackGame.setGameObjective(Data::IPG::Objectives::ZERORegrets_PlayerOne);
KnapsackGame.findNashEq();
std::cout << "The ZERO Regrets solution" << std::endl;
KnapsackGame.getX().at(0).print("Player 1:"); // Print the solution
KnapsackGame.getX().at(1).print("\n Player 2:");
} catch (ZEROException &e) {
throw ZEROException(e);
}
}
With the method setAlgorithm of
Game::IPG
, we set the algorithm to solve the Integer Programming Game. We can use eitherAlgorithms::IPG::CutAndPlay
to compute a mixed Nash equilibrium orAlgorithms::IPG::ZERORegrets
to compute the pure Nash equilibrium maximizing some function. In the latter case,The method setLCPAlgorithm specifies the algorithm used to solve the LCPs with the Cut-and-Play. It can be either
Data::LCP::Algorithms::MIP
,Data::LCP::Algorithms::PATH
, orData::LCP::Algorithms::MINLP
.The game’s objective (not supported by PATH) forces an objective into the LCP (Cut-and-Play) or MIP (ZERORegrets) problem as to increase the chances of finding a good equilibrium given the objective. In case
Algorithms::IPG::ZERORegrets
is selected, the algorithm will certify the optimality of the returned equilibrium.the Values can beData::IPG::Objectives::Quadratic
Data::IPG::Objectives::Linear
Data::IPG::Objectives::Feasibility
.Other options can be found in the documentation of
Game::IPG